Embark on a journey through the landscape of quality control and process improvement, where the concept of calculating dispersion unveils the subtle patterns and variations that shape the manufacturing canvas.
Embracing Diversity: Introduction to Dispersion
Before we dive into the calculations, let’s embrace the concept of dispersion. Picture it as the diverse strokes on an artist’s canvas, representing the spread or distribution of data points in a manufacturing process. Dispersion offers insights into the degree of variability, helping manufacturers understand the patterns that emerge within their datasets.
The Canvas of Variation: What Dispersion Reveals
Dispersion is like the canvas of variation, illustrating how data points are scattered or clustered. In quality control, it unveils the diversity among measurements, guiding manufacturers to comprehend the distribution of values. A tight dispersion suggests a more uniform process, while a broader dispersion indicates greater variability.
Understanding dispersion is crucial for manufacturers striving to enhance consistency and predictability in their processes.
The Brush Strokes: Calculating Dispersion
Now, let’s explore the brush strokes involved in calculating dispersion – a process that reveals the patterns and diversity within a dataset.
Step 1: Calculate the Mean – Finding the Center
Our journey commences with finding the center of the canvas – the mean (average) of the data points. Calculate the mean to establish the reference point around which the dispersion unfolds.
For example, if measuring the temperatures of a furnace, the mean temperature serves as the central value.
Step 2: Deviation from the Mean – Mapping the Patterns
Next, map the deviation of each data point from the mean. This step is akin to sketching the patterns on the canvas, showing how far each measurement deviates. Squaring these deviations ensures both positive and negative distances contribute to the overall dispersion.
In quality control, this step allows manufacturers to quantify the extent of variability within their datasets.
Step 3: Average Squared Deviations – Harmonizing the Palette
Calculate the average of the squared deviations. This step harmonizes the palette of dispersion, providing a comprehensive view of the average squared distance between each data point and the mean.
It’s like blending the colors on the canvas, capturing the overall level of variability in the manufacturing process.
Step 4: Square Root – Revealing the Artistry
The final touch is the square root of the average squared deviations. This step unveils the artistry within dispersion, returning to the original unit of measurement. It offers a clear and interpretable measure of how much individual data points typically deviate from the mean.
Dispersion becomes the canvas, showcasing the patterns and subtleties that define the variability in the manufacturing process.
Practical Insights: Applying Dispersion in Quality Control
Now that we’ve unraveled the calculations, let’s explore how dispersion provides practical insights into the realm of quality control.
Identifying Patterns: Tight Dispersion, Uniformity Prevails
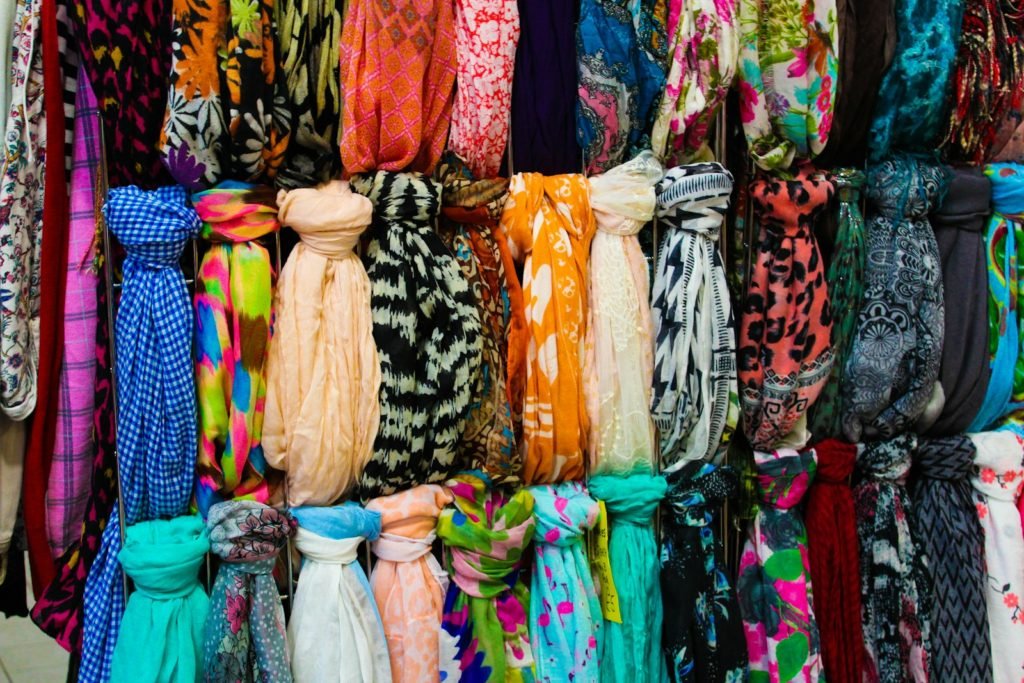
A tight dispersion indicates uniformity in the manufacturing canvas. If, for instance, monitoring the thickness of coatings, a tight dispersion suggests that most coatings have similar thicknesses. This signals a more controlled and predictable process, ensuring products meet desired specifications.
Identifying patterns through dispersion allows manufacturers to set quality standards and maintain consistency in their processes.
Detecting Variability: Broad Dispersion Alerts
Conversely, a broad dispersion acts as an alert for potential variability in the canvas. If monitoring the curing times of adhesives, a broad dispersion suggests significant differences in the time it takes for adhesives to cure. This alerts manufacturers to investigate potential factors contributing to variability and ensure process stability.
Detecting variability through dispersion enables manufacturers to address variations and maintain a more reliable manufacturing process.
Process Improvements: Analyzing Dispersion Trends
Dispersion can serve as a tool for analyzing trends in process improvements over time. If implementing changes to enhance efficiency, regularly analyzing dispersion allows manufacturers to assess whether the variation in the process has been reduced. A decreasing dispersion indicates a more controlled and stable manufacturing environment.
Analyzing dispersion trends provides valuable insights into the effectiveness of process improvements, guiding manufacturers toward continuous enhancement.
Challenges in the Palette: Navigating Dispersion Complexities
As with any artistic palette, calculating dispersion comes with its challenges. Let’s explore the hurdles manufacturers may face and how to navigate the complexities.
Sensitivity to Outliers: Disrupting the Canvas
Dispersion can be sensitive to outliers – data points that significantly deviate from the norm. Outliers can disrupt the canvas, influencing the squared deviations and potentially distorting the dispersion measure.
Manufacturers need to be mindful of outliers and may consider alternative measures of dispersion, such as the interquartile range (IQR), which is less sensitive to extreme values.
Interpretation of Values: Deciphering the Artistic Language
Interpreting the values of dispersion can be challenging. Since dispersion involves squared deviations, the resulting unit is not the same as the original data.
Manufacturers should communicate dispersion in an easily understandable way. For example, if product weights are in kilograms, the dispersion would be in square kilograms, which might be less intuitive. Providing clear explanations and using appropriate units can help decipher the artistic language of dispersion.
The Masterpiece of Improvement: Key Takeaways
As we conclude our journey through the artistry of calculating dispersion, let’s gather the key takeaways that will guide manufacturers in creating a masterpiece of continuous improvement.
Understanding Dispersion: A measure of the spread or distribution of data points, indicating the diversity within a manufacturing process.
Calculation Steps: Find the mean, measure deviation from the mean, square deviations, and average squared deviations, and take the square root to obtain a dispersion.
Practical Insights: Tight dispersion indicates uniformity, broad dispersion alerts to variability, and analyzing dispersion trends aids in process improvements.
Challenges: Sensitivity to outliers requires caution, and interpreting the unit of measurement may pose challenges in communication.
Crafting Artistry: Calculating Dispersion in Manufacturing
In the intricate canvas of quality control, calculating dispersion is the art of crafting artistry. Manufacturers, like skilled artists, must understand the patterns, variations, and subtleties within their datasets. Using dispersion as a tool, they can identify uniformity, detect variability, and continuously enhance the masterpiece of their manufacturing processes.