Embarking on a journey through the landscapes of quality control and process improvement, we find ourselves facing the challenges posed by skewed data and outliers. Let’s unravel the mysteries, exploring how these anomalies can disrupt the rhythm of manufacturing excellence.
The Symphony of Data
Before we delve into the complexities, let’s envision data as a symphony – a harmonious composition of information guiding the manufacturing orchestra. However, like any orchestra, data can encounter dissonance, with skewed data and outliers acting as the unexpected notes that disrupt the melody.
Skewed Data: The Unbalanced Tune
Imagine a musical composition where the majority of instruments play in a higher range, leaving only a few in the lower notes. This imbalance is akin to skewed data. In quality control, skewed data occurs when most values cluster towards one end of the scale, leaving a long tail on the other side.
Skewed data can mislead manufacturers, creating an illusion of the overall trend. For instance, if most products have similar dimensions but a few outliers are significantly larger, the average dimension may not accurately represent the majority.
Outliers: The Disruptive Crescendo
Now, consider a crescendo in a musical piece that suddenly rises above the expected volume. This unexpected surge is analogous to outliers – values that significantly differ from the majority. In quality control, outliers are data points that deviate significantly from the general pattern.
Outliers can skew analyses and lead to incorrect conclusions. For example, if monitoring production time and one machine consistently takes much longer than others, it can impact overall efficiency calculations.
Skewed Data: Unraveling the Imbalance
Let’s dive deeper into the world of skewed data, understanding its nuances and unraveling the impact on quality control.
Types of Skewness: The Uneven Flow
Skewedness comes in different forms, akin to different flows of a river. In positive skewness, the tail of the distribution points to the right, suggesting a few high values. In negative skewness, the tail extends to the left, indicating a concentration of low values.
Understanding the type of skewness is crucial for quality control. Positive skewness might suggest issues with the upper limit of a manufacturing process, while negative skewness could indicate challenges with the lower limit.
Impact on Averages: Distorted Central Tendency
Averages, like the central note in a melody, can be distorted by skewed data. In positively skewed distributions, the mean (average) is pulled towards the higher values, while in negatively skewed distributions, it’s influenced by lower values. This distortion can misrepresent the typical behavior of the manufacturing process.
Manufacturers must be cautious when relying on averages in the presence of skewed data. It might be more informative to use the median (middle value) as a measure of central tendency, as it is less affected by extreme values.
Misleading Interpretations: The Illusion of Trends
Skewed data can create illusions in trend analysis. For instance, if monitoring defect rates over time, a positively skewed distribution might make it seem like defects are decreasing when, in reality, a few high-value defects are skewing the perception.
Manufacturers need to be vigilant in interpreting trends, considering the influence of skewed data. Identifying the underlying causes of skewness is essential for making informed decisions in quality control.
Outliers: The Disruptive Notes
Now, let’s explore the disruptive notes of outliers, and understand their impact on the manufacturing melody.
Identification Challenges: Spotting the Odd Note
Identifying outliers can be akin to spotting an odd note in a complex musical composition. It requires keen observation and an understanding of the expected pattern. In quality control, outliers may represent defective products, unusual production times, or abnormal measurements.
Manufacturers must employ statistical techniques to identify outliers. Tools like box plots, scatter plots, or Z-scores can help highlight values that deviate significantly from the norm.
Impact on Variability: Disturbing the Harmony
Variability, like the natural fluctuations in a musical performance, is essential in manufacturing. Outliers, however, can disturb this harmony by introducing unexpected spikes in the data. For instance, if measuring the weight of products and an outlier represents an unusually heavy product, it can significantly impact the variability of weights.
Understanding the impact of outliers on variability is crucial for assessing the stability of manufacturing processes. Manufacturers may need to investigate and address the root causes of outliers to ensure consistent quality.
Influence on Decision-Making: Affecting the Crescendo
Outliers can sway decision-making, much like a sudden shift in the crescendo of a musical piece. If, for example, the production time for a critical component is consistently longer than expected, it might lead to delays in the entire manufacturing process.
Manufacturers should carefully evaluate the influence of outliers on decision-making. Consideration of the context, potential causes, and overall impact on the manufacturing flow is essential for making informed choices.
Navigating the Challenges: Strategies for Quality Control
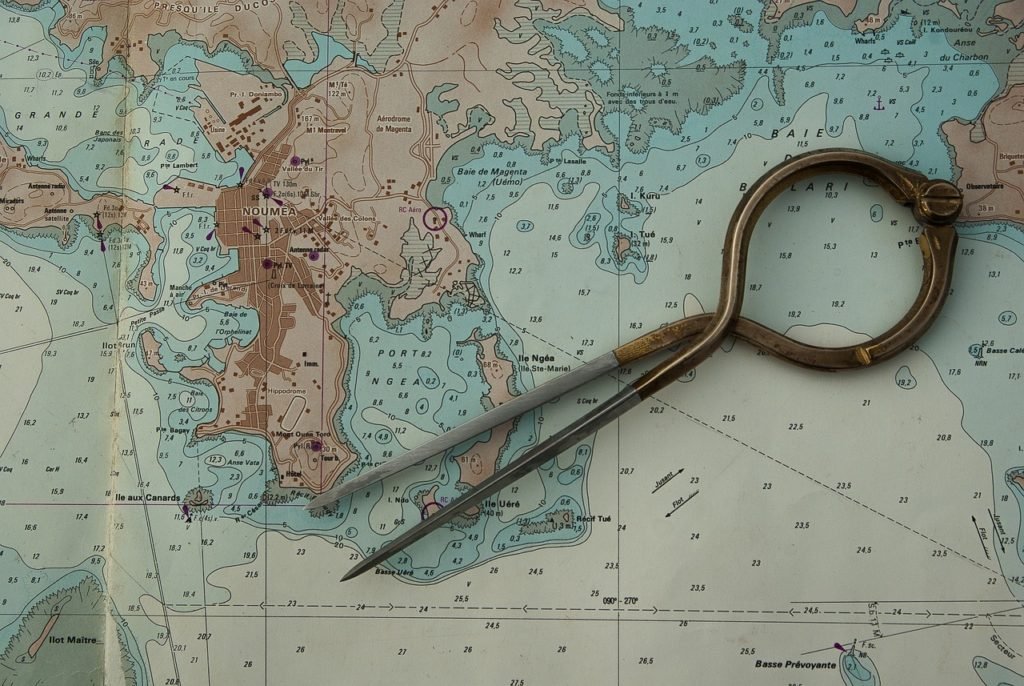
As we navigate the challenges posed by skewed data and outliers, let’s explore strategies for maintaining the harmony of quality control.
Transformation Techniques: Reshaping the Melody
Just as musicians use techniques to reshape a melody, manufacturers can employ transformation techniques to address skewed data. Logarithmic transformations or power transformations can help mitigate the impact of skewness, creating a more symmetrical distribution for analysis.
Transformation techniques should be applied judiciously, considering the characteristics of the data and the objectives of quality control. They can be a valuable tool in restoring balance to the manufacturing melody.
Robust Measures: Fortifying Against Disruption
To fortify against the disruptive influence of outliers, manufacturers can rely on robust measures. Median, interquartile range (IQR), or median absolute deviation (MAD) are less sensitive to extreme values compared to mean and standard deviation.
Using robust measures ensures that outliers have less influence on the overall analysis. Manufacturers can maintain a more stable baseline for quality control, minimizing the impact of extreme values on decision-making.
Root Cause Analysis: Unveiling the Source
Just as a musician seeks the source of a discordant note, manufacturers must conduct root cause analysis to unveil the source of outliers. Understanding why an outlier occurs – whether it’s a machine malfunction, a material defect, or a process anomaly – is crucial for implementing targeted improvements.
Root cause analysis helps manufacturers address underlying issues, preventing the recurrence of outliers. It’s a proactive approach to quality control that focuses on continuous improvement.
Monitoring Trends: Staying in Tune
To mitigate the impact of skewed data and outliers on trend analysis, manufacturers should implement vigilant monitoring. Regularly assessing the distribution of data, identifying shifts in patterns, and understanding the dynamics of skewness can help maintain a more accurate interpretation of trends.
Monitoring trends ensures that manufacturers stay in tune with the evolving nature of their processes. It allows for timely adjustments and interventions to address emerging challenges.
The Ongoing Symphony: Key Takeaways
As we conclude our exploration of the effects of skewed data and outliers in quality control, let’s gather the key takeaways that will guide manufacturers in orchestrating an ongoing symphony of improvement.
Skewed Data: Understanding types of skewness and their impact on averages and trend analysis.
Outliers: Recognizing the challenges in identifying outliers and their influence on variability and decision-making.
Transformation Techniques: Using logarithmic or power transformations to address skewed data.
Robust Measures: Employing median, IQR, or MAD for more stable analyses in the presence of outliers.
Root Cause Analysis: Conducting in-depth analysis to unveil the source of outliers and address underlying issues.
Monitoring Trends: Regularly assessing data distributions to stay in tune with the dynamics of manufacturing processes.
Harmony Restored: Navigating Anomalies in Quality Control
In the grand symphony of quality control, manufacturers navigate the challenges of skewed data and outliers with the finesse of conductors. It’s not just about recognizing the unexpected notes but orchestrating strategies to restore harmony and maintain the rhythm of continuous improvement.
Understanding the effects of skewed data and outliers is pivotal for manufacturers striving for excellence. Like skilled musicians, they must be attuned to the nuances, leveraging techniques and measures that ensure the ongoing symphony of quality control remains melodious and refined.